When file size is restricted, humans beat traditional algorithms at representing images, research finds.
Sending a link instead of uploading a massive image is one trick humans use to convey information without burning through data. It and other tricks might inspire an entirely new class of image compression algorithms, according to research by a team of Stanford University engineers and high school students.
The researchers asked people to compare images produced by a traditional compression algorithm that shrink huge images into pixilated blurs to those humans created in data-restricted conditions—text-only communication, which could include links to public images. In many cases, the products of human-powered image sharing proved more satisfactory than the algorithm’s work. The researchers will present their work March 28 at the 2019 Data Compression Conference.
“Almost every image compressor we have today is evaluated using metrics that don’t necessarily represent what humans value in an image,” says Irena Fischer-Hwang, a graduate student in electrical engineering at Stanford University and coauthor of the paper. “It turns out our algorithms have a long way to go and can learn a lot from the way humans share information.”
The project results from a collaboration between researchers led by Tsachy Weissman, professor of electrical engineering, and three high school students who interned in his lab.
“Honestly, we came into this collaboration aiming to give the students something that wouldn’t distract too much from ongoing research,” says Weissman. “But they wanted to do more, and that chutzpah led to a paper and a whole new research thrust for the group.”
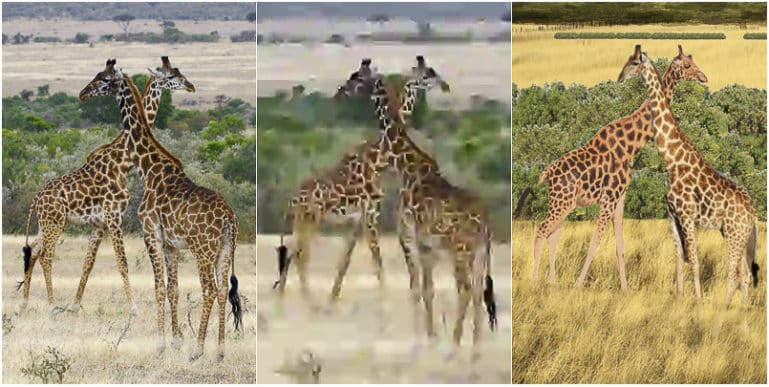
Reconstructing images
Converting images into a compressed format, such as a JPEG, makes them significantly smaller, but loses some detail—this form of conversion is often called “lossy” for that reason. The resulting image is lower quality because the algorithm has to sacrifice details about color and luminance in order to consume less data. Although the algorithms retain enough detail for most cases, Weissman’s interns thought they could do better.
In their experiments, two students worked together remotely to recreate images using free photo editing software and public images from the internet. One person in the pair had the reference image and guided the second person in reconstructing the photo. Both people could see the reconstruction in progress but the describer could only communicate over text while listening to their partner speaking.
The eventual file size of the reconstructed image was the compressed size of the text messages sent by the describer because that’s what would be required to recreate that image. (The group didn’t include audio information.)
The students then pitted the human reconstructions against machine-compressed images with file sizes that equaled those of reconstruction text files. So, if a human team created an image with only 2 kilobytes of text, they compressed the original file to the same size. With access to the original images, 100 people outside the experiments rated the human reconstruction better than the machine-based compression on 10 out of 13 images.
Blurry faces
When the original images closely matched public images on the internet, such as a street intersection, the human-made reconstructions performed particularly well. Even the reconstructions that combined various images often did well, except in cases that featured human faces. The researchers didn’t ask their judges to explain their ranking but they have some ideas about the disparities they found.
“In some scenarios, like nature scenes, people didn’t mind if the trees were a little different or the giraffe was a different giraffe. They cared more that the image wasn’t blurry, which means traditional compression ranked lower,” says Shubham Chandak, a graduate student in Weissman’s group and coauthor of the paper. “But for human faces, people would rather have the same face even if it’s blurry.”
High schoolers vs. the algorithm
This apparent weakness in the human-based image sharing would improve as more people upload images of themselves to the internet. The researchers are also teaming up with a police sketch artist to see how his expertise might make a difference. Even though this work shows the value of human input, the researchers would eventually try to automate the process.
“Machine learning is working on bits and parts of this, and hopefully we can get them working together soon,” says Kedar Tatwawadi, a graduate student in Weissman’s group and coauthor of the paper. “It seems like a practical compressor that works with this kind of ideology is not very far away.”
Weissman stresses the value of the high school students’ contribution, even beyond this paper.
“Tens if not hundreds of thousands of human engineering hours went into designing an algorithm that three high schoolers came and kicked its butt,” says Weissman. “It’s humbling to consider how far we are in our engineering.”
Lead authors of this paper are Ashutosh Bhown of Palo Alto High School, Soham Mukherjee of Monta Vista High School, and Sean Yang of Saint Francis High School.
Funding came from the National Science Foundation, the National Institutes of Health, the Stanford Compression Forum, and Google.
Source: Stanford University