Machine learning powers a new kind of drone flight controller software, researchers report.
After Wil Koch flew a friend’s drone for the first time, operating it through “first-person view” where a person wears a headset connected to a video feed streaming live from a camera on the drone, he thought it was amazing. So amazing that he went out that same day and purchased his own system—a video headset, controller, and quadcopter drone, named for the four propellers that power it.
“You put the goggles on and they allow you to see live video transmitting from a camera mount on the drone,” Koch says. It is “by far, the coolest thing.”
First-person-view drone racing is gaining popularity among technology enthusiasts, and there are competitive races around the world. Just a few weeks after his introduction to the sport, Koch, a graduate researcher at the Rafik B. Hariri Institute for Computing and Computational Science at Boston University, founded Boston Drone Racing as a new computing club.
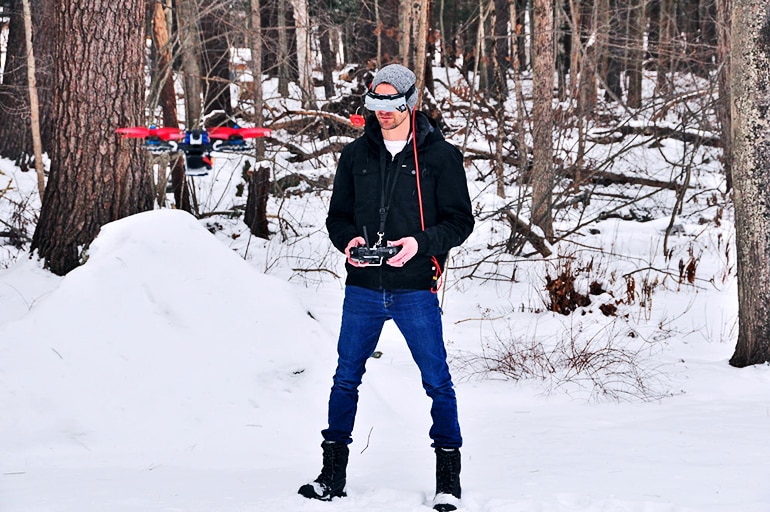
But because Koch thinks like a computer scientist, his mind soon turned to looking for ways that he could take “the coolest thing” and make it even cooler. What if, he wondered, you could leverage artificial intelligence to fly a drone faster and more precisely than the standard set-up?
Ditching ‘zombies’ for drones
Koch probably would never have pursued the idea if not for that day when he flew his friend’s drone from a bird’s-eye view. But it was his newfound passion that would inspire a breakthrough in neural network technology, when he and a team of collaborators built Neuroflight to optimize flight performance.
“[His] prior love was related to cybersecurity and defending against autonomous cyberattacks from ‘zombie’ computers,” says Koch’s faculty advisor Azer Bestavros, founding director of the Hariri Institute and senior author of the team’s first public paper describing Neuroflight.
But after Koch fell in love with drone racing, “he flipped on me,” Bestavros says with a laugh. Looking into research at the intersection of drones and artificial intelligence, Koch and Bestavros learned that General Electric and other industry titans were aggressively pursuing technology in that space.
“Wil and I confirmed the value and potential of this line of work, thinking about control of autonomous vehicles and how you might use AI and machine learning to do that,” says Bestavros, who is also a professor of computer science at the College of Arts & Sciences.
“Just like the progression of technology in Formula 1 racing has created technologies we see in our own vehicles,” he says their hope is that developing new solutions that withstand the extremes of drone racing will push the broader field of autonomous flight technology to a better place.
A better controller
Currently, drones and most other remote-controlled vehicles are operated through linear controllers that can’t adapt to changing conditions.
“Imagine you’re driving a car on the road and one tire goes flat,” Bestavros says. “You, as the driver, wouldn’t do the same things you would do if you were driving the car with all four wheels. You’d steer and accelerate differently.”
A typical quadcopter uses a conventional controller—known as a proportional integral derivative, or PID, controller in the computer science world. That allows the operator to give the drone commands to move in a certain direction and velocity by moving the controller’s joysticks. But current controller technology doesn’t contain any inherent ability to adapt to changing conditions, like higher winds or (hopefully not) even the loss of a propeller.
The Neuroflight controller, Koch says, is trained in computer simulation to adapt to a wide range of different events, correcting the drone’s position inside a dynamic and changing, albeit digital, environment. After simulation training, the “educated” neural network goes to work in the real world by sending signals to the drone motors, telling them how to respond so that the quadcopter moves in the exact way that its operator intends.
“PID is a linear control system, but the environment is nonlinear,” says Koch, who is a College of Arts & Sciences graduate student in computer science. “We’re ripping out that PID controller and dropping in a trained neural network.”
What’s next?
After three months of experimental computer simulation, Neuroflight took to the skies on its maiden voyage in November 2018, a milestone for the world’s first machine-learning-optimized drone controller. The drone racing community is already buzzing about the news, with online influencers highlighting the advance and breaking down how the technology works on their YouTube channels.
“Up until now, our primary goals were to identify if this was possible or feasible,” Koch says. That “it got off the ground and works in the real world” is important in itself. But he’s quick to add: “We haven’t even begun the most important aspects of making Neuroflight more powerful than PID. At the moment, this is not something I would take to a race; it still needs attention and work to address particular things such as drift,” which can cause a drone to veer off course.
To take Neuroflight to the next level, Koch is working on building a digital twin of his racing drone.
“We’ve been training Neuroflight in simulation using a completely different aircraft,” Koch says. “Now that we have a baseline, our goal is to reduce that gap between simulation and real world, and the first step in doing that is to create a complete digital replica of the real drone.”
Koch will lean on his CAD software skills to build 3D models and assemble his drone’s components in a computer-simulation environment. The more complicated step, he says, will be creating the dynamic models for the drone’s motors and propellers.
“The neural network shouldn’t be able to tell the difference between simulated and real world. That’s why we’re building up the digital twin concept,” he says. “Our hypothesis is that since we’re going to be increasing the realism in simulation, we should see increases in accuracy in the real world.”
The continuing work, Koch and Bestavros say, will showcase the true benefits of using machine learning for control, improving effectiveness in the face of uncertainty.
“What Wil is doing is part of the broader picture of using AI-controlled technology,” as well as advancing human acceptance of AI controllers, Bestavros says. To move basic research in this space forward, he says, “drones are easy because they aren’t carrying human beings.”
Source: Boston University