Researchers are using big data to determine the potential impact of demographic diversity on new knowledge in the field of history.
Diverse teams are often associated with rapid discovery, yet few studies have examined whether and to what extent diversity in demographics, such as an individual’s gender and race, leads to new ideas and knowledge. “These questions are part of a longstanding discussion in the research community concerning who creates knowledge and the knowledge produced,” says Londa Schiebinger, professor of history of science at Stanford University.
Schiebinger and her colleagues’ latest work in this arena, published in PLOS ONE, used big data and computer modeling to map how women and men have contributed to developments and breakthroughs in the field of history over time between the years 1950-2015.
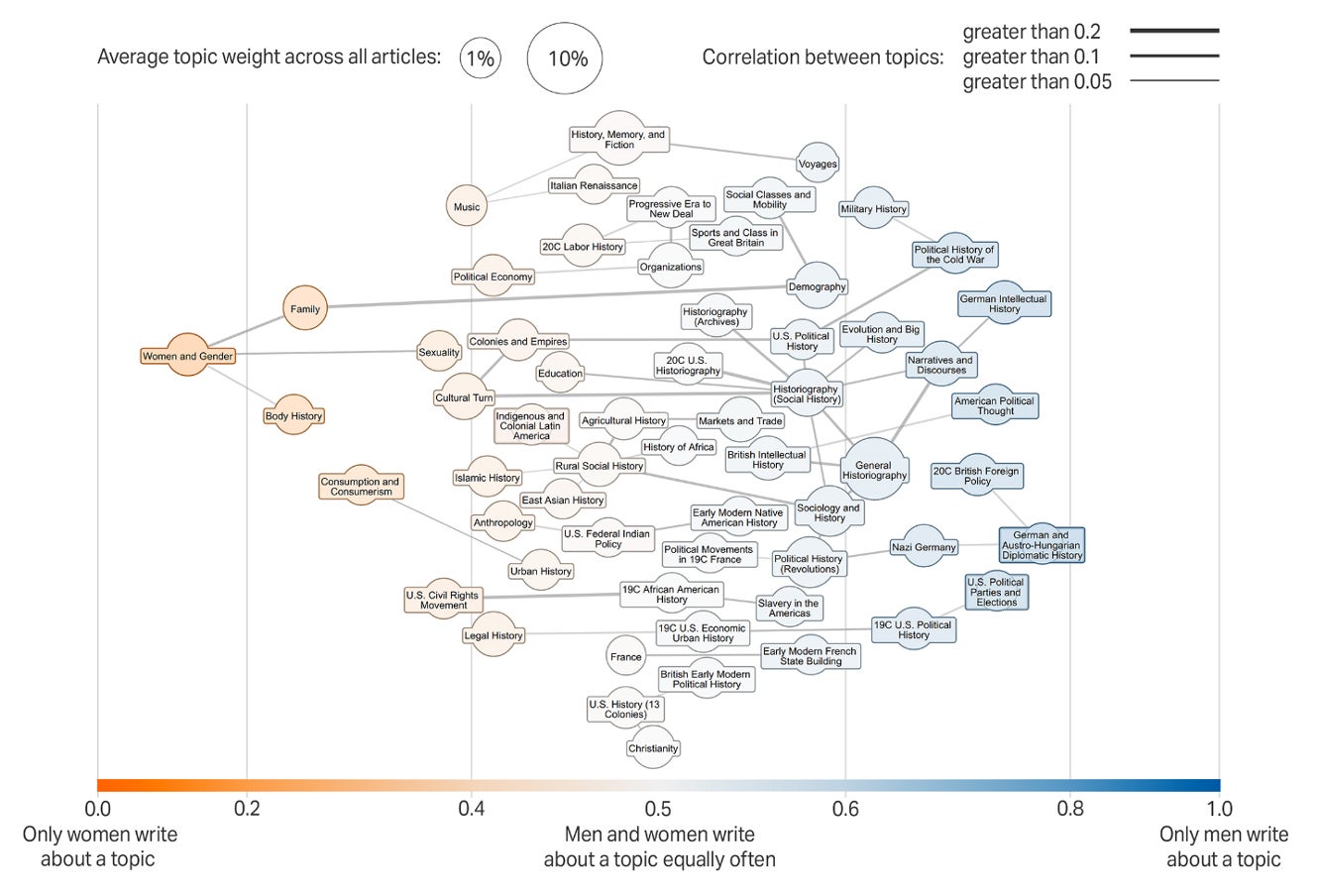
Here, two of the paper’s nine coauthors, senior author Schiebinger, who is also director of Stanford’s Gendered Innovations in Science, Health & Medicine, Engineering, and Environment Project, and Mathias W. Nielsen, formerly a Gendered Innovations postdoctoral scholar at Stanford, now an associate professor of the sociology of science at the University of Copenhagen, discuss the study and how increased gender diversity in research affects not only the scientists doing the work but also what research is taking place:
What prompted this study and what’s the broader context of this research?
Schiebinger: Too often diversity, equity, and inclusion (DEI) means demographic diversity—or the diversity of bodies in the room. What we are interested in is intellectual diversity—or the production of new knowledge. Does demographic diversity result in new knowledge? Do newcomers launch new areas of research? What new knowledge is produced? This is what motivated this study.
Nielsen: A few years ago, Londa, Carter Walter Bloch of Aarhus University in Denmark, and I conducted a review of the literature on gender diversity in science that identified three types of diversity: diversity in teams, diversity in methods, and diversity in research questions. At that point, gender diversity was commonly used to refer to the composition of teams. That motivated us to study changes in researcher demographics and research agendas within the field of history over time.
How is this research different from other studies of diversity and discovery?
Nielsen: A few prior studies have examined associations between researcher demographics (such as gender and nationality) and research topics (primarily in the social sciences and medical sciences) at a single point in time. Our study traces developments in gender diversity and research questions over time enabling us to tease out how the field of history changed as women entered the discipline.
Schiebinger: When newcomers enter an academic field, do they create new knowledge and open new areas of research? … They are not questions we could answer using traditional historical methods. Funding from the Stanford Institute for Human-Centered Artificial Intelligence (HAI) allowed collaboration between historians and natural language processing experts including lead author Stephan Risi of Massachusetts Institute of Technology; Stanford Graduate School of Education (GSE) graduate students Lanu Kim and Emma Kerr; Emer Brady of Aarhus University in Denmark; Daniel McFarland, professor in GSE; Dan Jurafsky, the Jackson Eli Reynolds Professor in Humanities and a professor of computer science; and James Zou, assistant professor of biomedical data science.
How did your team use big data and modeling to uncover how the field of history changed when women entered this discipline?
Schiebinger: The first step was getting the data—which took about a year. In the end, JSTOR generously assisted us with the data we needed. We inferred author gender using Social Security Administration data, computer algorithms, and hand labeling to identify how much women and men contribute to each topic. Note that this study uses gender inference as a way of analyzing large-scale dynamics, not to determine an individual’s gender.
Nielsen: We used the text from history PhD dissertations published in the US between 1980-2015 and history articles published in US journals between 1950-2015 to identify and explore what research questions have occupied men and women historians. For the analysis, we used correlated topic modeling—a form of computational text analysis—to generate a large list of research topics and to identify the most prevalent topics in each publication.
What did you find?
Schiebinger: In the field of history, men and women explored many of the same topics, including religion, colonialism, African history, and aspects of historiography. Yet, there is a notable divergence. Men tended to focus on topics related to political and intellectual history, military history and the study of history on a large scale (aka “Big History”). Women historians tended to write about topics related to gender, body history, patterns of consumption, family and households, sexuality, the US civil rights movement, and the cultural turn. Our analysis demonstrates that while women pioneered topics such as gender and women’s history or the history of sexuality, these topics broaden over time to become areas that women and men historians widely embraced.
What are some of the limitations, potential applications, and future directions of this research?
Nielsen: There is a “chicken-and-egg problem.” Which came first, the openness of disciplines to new questions or the increasing participation of underrepresented groups in those disciplines? Presumably, the relationship is reciprocal, but the direction of causality is difficult to establish, even in large-scale, multi-year studies like ours.
Our study demonstrates a clear link between researcher demographics and the questions raised in historical research. Our findings suggest that increased diversity can broaden the repertoire of perspectives, values, and questions at play in academic fields. I hope to see much more research on this important question in the future.
Schiebinger: Our work has three limitations and ethical concerns. First, current name algorithmic tools do not provide categories beyond the binary “woman” or “man.” Second, we found no satisfactory tools to classify authors’ ethnicity. This resulted in our third limitation—we could not analyze how gender, ethnicity, and other social categories might interact. We could not see, for example, the distinctive contributions of Black women, of which there are many, including the development of the concept of intersectionality itself.
The categories of race and gender are currently undergoing massive conceptual change and this work has the potential to support social change and interventions. For example, the National Institutes of Health recently tasked the National Academies of Sciences to develop new gender categories for patient records, birth certificates, and the like. This, in turn, will create new datasets for the type of social analyses we demonstrated in our analysis of history. Our ultimate goal is to work across the social sciences, humanities, and artificial intelligence to develop robust tools for intersectional approaches to gender, race, and ethnicity in knowledge production.
Source: Stanford University